Community Acquired Pneumonia and COVID-19 Artificial Intelligence (AI) Predictive Engine (CAPE) is an AI-enabled tool that can predict the severity of pneumonia in patients, including COVID-19 patients, based on a chest x-ray image. This Smart Health AI predictive engine enables closer monitoring and treatment of patients with severe pneumonia for improved patient outcomes through timely triaging and treatment.
CAPE was co-developed by Synapxe and a multi-disciplinary team at Changi General Hospital (CGH), one of several public healthcare institutions searching for ways to fortify their preparations and responses when COVID-19 first reached Singapore.
About Community Acquired Pneumonia and COVID-19 Artificial Intelligence (AI) Predictive Engine (CAPE)
CAPE at a glance
Opportunity
Pneumonia is one of the leading causes of death worldwide, and the main cause of deterioration in COVID-19. As pneumonia severity correlates to the degree of Chest X-Ray (CXR) lung image abnormality, CGH’s Respiratory and Critical Care Medicine and Radiology teams recognised the potential in leveraging AI to predict the severity of pneumonia from CXR images and worked with Synapxe's Data Analytics & Ai (DNA) team to develop CAPE.
Solution
CAPE utilises both radiology images and electronic medical records data for a single end-to-end deep learning model. Using more than 3,000 CXR images and 200,000 data points, CAPE was trained to generate a score based on indicators of pneumonia severity. Initial validation tests at CGH shows that CAPE has an approximate accuracy of 80 per cent in predicting severe pneumonia, comparable to traditional pneumonia severity tools that are scored manually.
Impact
The risk score generated by CAPE can serve as a decision support for doctors. Patients who are likely to require critical care can be closely monitored and receive treatment in a timely manner. The ability to quickly predict the patient’s expected pneumonia severity enables clinicians and administrators to efficiently allocate healthcare resources, where there may be an increased need for inpatient care and critical care support.
Future Outlook
Beyond local healthcare settings, CAPE can potentially be calibrated to identify and predict the severity of respiratory infections globally.
The CGH team is working to validate the model in other public health institutions in Singapore to improve the robustness of the model. The team is also exploring collaborative models, including uploading it as a “freeware” collaborative tool on a research platform for interested researchers.
The CGH team is working to validate the model in other public health institutions in Singapore to improve the robustness of the model. The team is also exploring collaborative models, including uploading it as a “freeware” collaborative tool on a research platform for interested researchers.
Resources
KDD: AI prognostication tool for severe community-acquired pneumonia and covid-19 respiratory infections
Read more

IEEE: Ensemble image explainable AI (XAI) algorithm for severe community-acquired pneumonia and COVID-19 respiratory infections
Read more

BMJ: Chest radiograph-based artificial intelligence predictive model for mortality in community-acquired pneumonia
Read more

Case Study
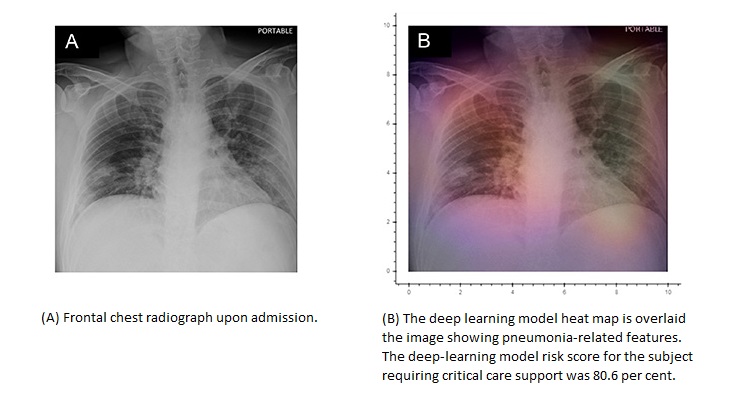
A 42-year-old man was tested positive for COVID-19 and CAPE was able to predict the need for critical care support to be at 80.6 per cent. The patient was subsequently admitted to ICU for deteriorating oxygen levels.
Partner
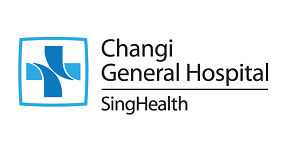